Blog
Intelligence
How Data Science Helps Marketers Mine for Truth
In case you haven’t noticed, we’re living in the Information Age. Have a question? Google’s got the answer. But in a world with so much information, it can be extremely difficult to cut through the noise and evaluate the definition of truth. From a micro to macro level, having reliable, researched-based information is the foundation of success for any objective.
Cue data science and all its glory.
An interdisciplinary field, data science has become increasingly vital in today's technological revolution and the evolution of artificial intelligence. It uses scientific methods, algorithms and systems to extract knowledge from structured and unstructured data, benefitting almost every industry on the planet. From banking to healthcare, data science looks at not only what people want, but what they need. And as marketers, we see opportunity from a mile away. If we can understand our audience better, we can market to them more meaningfully.
Let’s take a deep dive into how data science works.
The Process
Collect
Collecting the right data is key. It's foundational and crucial to success: Determine what information you're seeking and what answers you're looking to solve, then let those questions guide which data points you'll need to collect.
Cleanse
Once the data is collected, it needs to be cleansed. Real-life data is almost always messy. So cleaning it might mean filling in missing values and correcting misspellings and formatting.
Explore
Next, explore the data to see what's in its fields. This step will typically take longer, but getting to know the data will make "modeling" it much easier. You might realize you're missing some necessary fields to answer your central questions, or that the data you do have isn’t usable. This is where the iterative process of data science comes into play. It’s common to get to this stage and then have to loop back to the first two phases to get the data in the right shape to model.
Model
Now it's time to build and run data models. You'll want to try different iterations of the model to see which performs best while still making logical sense. This step involves a bit of trial and error, as well as a lot of evaluation against both your data and other models.
Communicate
Once you have a model that performs well, the next step is to communicate your findings to your stakeholders. This usually involves visualizations of the data and exploration discoveries, as well as presenting the model and its performance. From here, you'll need to move the model into production so it can be used and monitored in real time. Communication during this phase is important so you can highlight how hard your data has worked to gather the insights your stakeholders need to make informed business decisions.
Remember, it's not a linear process
You might get to the “explore” phase and realize you need more or different types of data. This is normal. You'll just need to go back to the “collect” phase to ensure you're gathering the right input based on the questions you need to answer. This is a highly iterative process and will vary based on the nature of your project.
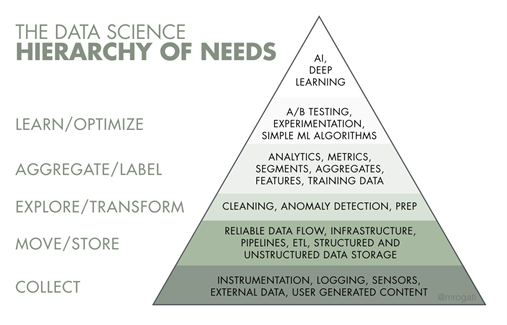
The Stages
After going over the general data science process, it’s time to look at the stages of data science. These build on each other and have similar names to the data science process because they resemble one another. You need these building blocks to go through the process. The hierarchy starts at collect—the most important piece of all of data science. Because being able to collect usable data helps tell a story.
Once a form of collection is up and running, you need a place to store the data—and maybe even be able to set up a flow to continuously collect and store data as you obtain more of it. From there, you'll need a process to clean and validate the data to ensure its accuracy.
Once those steps are completed, the real fun begins! You can now use the data to perform descriptive analytics, which just means reporting on what's already happened. Then you'll advance to prescriptive analytics, which means using past data to predict future outcomes.
Data scientists spend 80% of their time cleaning and manipulating data, and only 20% of their time actually analyzing it.
Getting from descriptive to prescriptive analytics is no easy task. You need a solid foundation for your collect, store and clean processes, as well as the tools and statistical knowledge to run, diagnose and improve data models. Ideally, once those are in a good place, you can introduce artificial intelligence (AI) and deep learning to glean even more insights from the data.
Having a solid information base is crucial in progressing up the data science pyramid. While data science is mostly about collecting, storing and cleansing data, the modeling and predictive analytics are the reward for doing all of that well.
Data doesn't lie
We know it can be a lot to digest. Extracting information that tells an honest story is the very reason why this discipline exists. While we're big proponents of marketing with empathy, we also leverage data intelligence at Dixon Schwabl to inform our marketing programs and guide our clients with facts, trends and projections that drive their business forward.
Emily Ferry
Emily Ferry is DS+CO’s media coordinator, keeping projects on track and campaigns on target.